Network-based prediction of drug combinations
Nature communication 2019 / 03 IF=15
https://doi.org/10.1038/s41467-019-09186-x
![]()
文章使用数据
1、PPI
high-quality protein-protein interactions (PPIs)
原文说有243,603 PPIs connecting 16,677 unique proteins ,但是根据文章附件链接只有217160 PPIs, 涉及15970个蛋白质
2、药物数据
(1)药物组方:DCDB
1,363 clinically reported drug combinations for 904 distinctive components
http://public.synergylab.cn/dcdb/index.jsf
在本文,作者仅关注 pairwise drug combinations :681 combinations connnecting 362 drugs
(2)TTD数据库
drug, target, and drug-target pathway information
3、疾病靶点
(1)OMIM:The OMIM database (Online Mendelian Inheritance in Man)
literature-curated human disease genes with various high-quality experimental evidences.
(2)CTD:The Comparative Toxicogenomics Database
only manually curated gene-disease interactions from the literatures were used.
(3)ClinVar:relationships among sequence variation and various human phenotypes
https://www.ncbi.nlm.nih.gov/clinvar/
cardiovascular[Disease/Phenotype]
(4)GWAS
unbiased SNP-disease associations with genome-wide significance
p <5.0×10 -8
(5)GWASdb
http://jjwanglab.org/gwasdb NOT USED
SNP-trait associations from GWAS for PubMed and other resource
p <1.0×10 -3
(6)PheWAS Catalog
https://phewascatalog.org/phewas
phewas.mc.vanderbilt.edu
SNP-trait associations identified by the phenome-wide association study (PheWAS) paradigm within electronic medical records
p <0.05
(7)HuGE Navigator
https://phgkb.cdc.gov/PHGKB/phgHome.action?action=home
an integrated disease candidate gene database based on the core data from PubMed abstracts using text mining algorithms
literature-reported disease-gene annotation data with known PubMed IDs
(8)DisGeNET
16,000 genes and 13,000 diseases
only expert-curated data
附件上传的数据
The publicly available human protein–protein interactome (Supplementary Data 1)
experimentally validated drug–target interactions (Supplementary Data 2)
experimentally validated drug combinations (Supplementary Data 3)
分析思路
1、药物靶点群与疾病群距离
-
假设:Disease proteins are not scattered randomly in the interactome, but tend to form localized neighborhoods, known as disease modules
-
计算药物与疾病的Network-based proximity
$$ d(X,Y) = \frac{1}{||Y||}\sum_{y\in{Y}}min_{x\in{X}}d(x,y) $$
- 计算相同数量size与连接度degree的靶点群与疾病靶点群的距离,拟合高斯分布,进行Z值转换。由此判断药物距离与疾病距离是否足够近(z<0)。 $$ z = \frac{d-\mu}{\sigma} $$
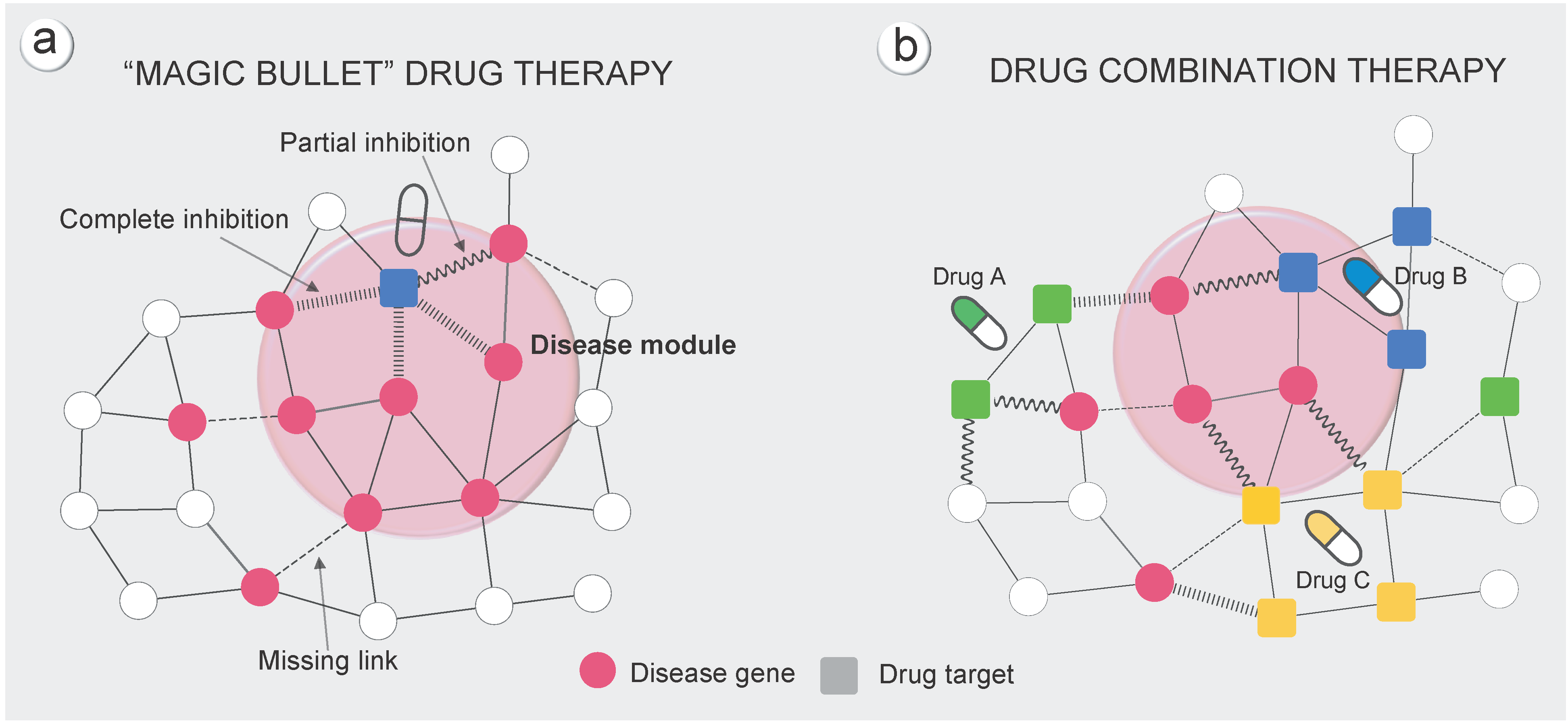
2、计算药物靶点群与药物靶点群的距离
- 药物靶点的数目通常比较少(FDA批准的1978个药物中,平均靶点为3。由上公式计算的药物与药物间距离的随机化不符合正态分布。
- 进一步改进计算公式,考虑两个药物的影响范围。同第一点,进行Z值转换。
$$ s_{AB} = d_{AB} - \frac{d_{AA} + d_{BB}}{2} $$
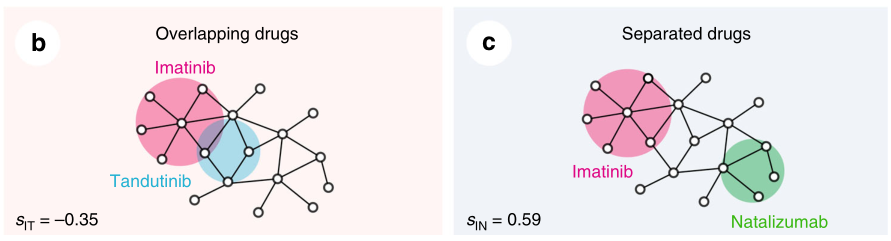
通过药物组合实验数据、药物性质以及药物距离的关系
(1)两个药物靶模块之间的拓扑关系也反映了生物和药理学关系。
(2)FDA批准的两两药物组合之间的距离比较近。如下图A
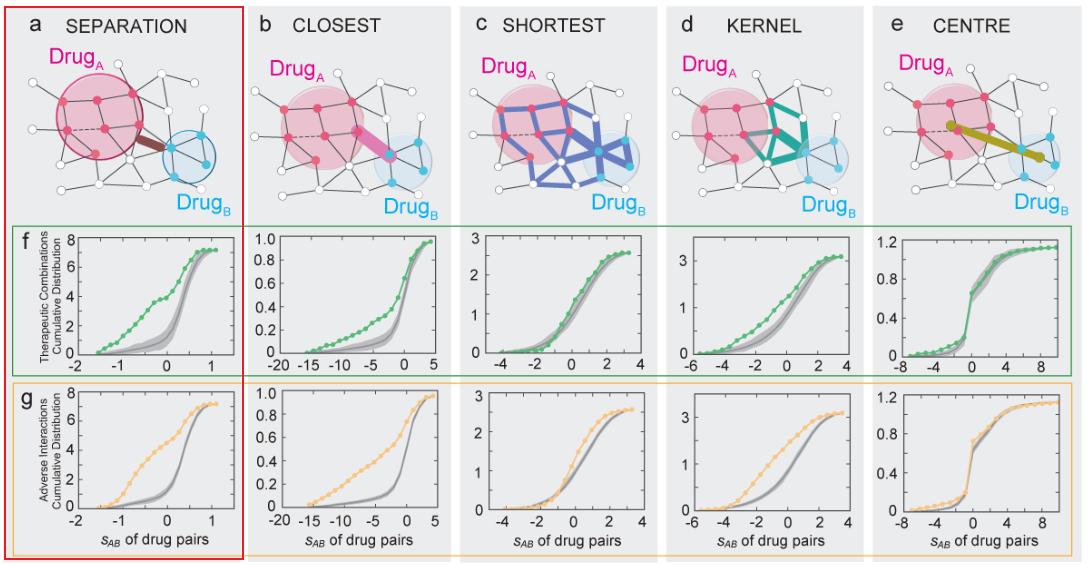
3、药物-药物-靶点群关系
- 文章分为6种药物-药物-靶点群关系
- 结合已知药物组合数据发现:only drug pairs that have Complementary Exposure relationship to the disease module(下图B) show a statistically significant efficacy for drug combination therapies
- 最后文章利用Complementary Exposure relationship,预测、筛选具有抗高血压的药物组合。发现预测显著的结果有相应的研究数据支持,表明这种药物组合发现模式的准确性。
前期准备好数据很重要。不仅仅是用于预测的数据,还要有支持验证的数据。